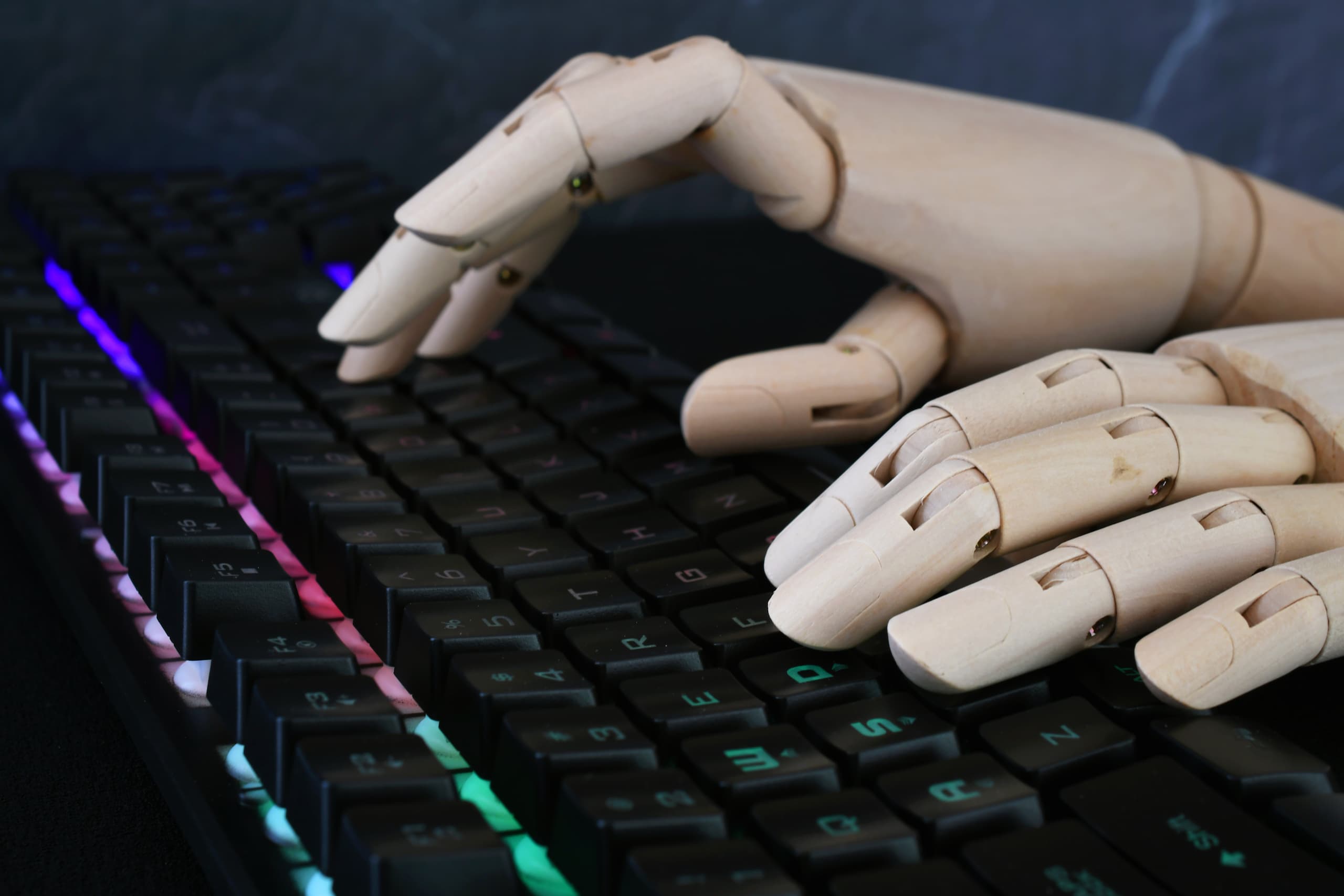
The advancement of machine learning has revolutionized the way companies manage and analyze large volumes of data. This approach not only allows for automating complex tasks but also identifying hidden patterns, generating more accurate predictions, and improving decision-making. In a world where information is the most valuable resource, integrating machine learning in data management is not an option but a necessity for any company that wants to remain competitive. In this blog, we will explore how machine learning is transforming data management and why it is crucial for the future of analysis.
What is Machine Learning Applied to Data Management?
Machine learning is a branch of artificial intelligence that allows systems to learn from data without being explicitly programmed. In the context of data management, machine learning is used to automate analysis processes, improve data quality, and extract valuable insights that might otherwise go unnoticed.
Instead of relying on predefined rules, machine learning algorithms are trained on historical data to identify patterns, classify information, and make future predictions. This continuous learning capability improves the accuracy and efficiency of data analysis as more data becomes available.
According to a McKinsey report, 63% of companies using machine learning in data management report a significant improvement in strategic decision-making. This type of technology enables organizations to better understand their information and make more accurate decisions in real time.
Benefits of Machine Learning in Data Management
1. Improving Data Quality
One of the main challenges companies face is maintaining data quality. Incomplete, duplicated, or incorrect data can lead to unreliable analysis and poor decision-making. Machine learning enables the identification and correction of data errors automatically. Algorithms can detect anomalous patterns, correct common errors, and deduplicate records in large datasets.
For example, if a company stores customer records and some contain errors in address or phone fields, machine learning models can identify these discrepancies and suggest corrections based on previous behavior patterns. A Forrester report shows that companies using machine learning for data cleansing improve their database quality by 40%.
2. Predictive Analysis and Decision-Making
Predictive analysis is one of the most powerful applications of machine learning in data management. By analyzing large volumes of historical data, algorithms can predict future behaviors with a high degree of accuracy. This is especially useful in areas such as demand forecasting, customer segmentation, and risk identification.
For example, a retailer can use machine learning to analyze customer purchasing patterns and predict which products will be more popular in upcoming seasons. This way, they can adjust their inventory and marketing strategies more effectively. A Deloitte study reveals that companies implementing machine learning-based predictive analysis increase their revenue by 20% by making more informed and timely decisions.
3. Process Automation
Process automation is another key benefit of machine learning in data management. Algorithms can perform repetitive tasks more quickly and efficiently than humans, freeing up time and resources for more strategic tasks. This includes data classification, report generation, and the analysis of large volumes of information in real time.
For example, in the financial sector, machine learning algorithms can analyze thousands of transactions in seconds, detecting suspicious behavior and potential fraud. Similarly, in marketing, machine learning can help automate the segmentation of advertising campaigns, ensuring that the message reaches the right audience at the right time.
A PwC report indicates that 45% of companies that have implemented machine learning have managed to reduce the time dedicated to routine tasks by 30%. Automation not only improves efficiency but also allows teams to focus on more complex analyzes and strategic decisions.
Applications of Machine Learning in Data Management
1. Data Cleaning and Purging
Machine learning can help address one of the most common issues in data management: data cleaning and purging. Algorithms can detect outliers, eliminate duplicates, and correct erroneous data automatically. Additionally, they can identify patterns in incomplete data and suggest precise values to fill empty fields.
For example, if you have a customer database with incomplete records, machine learning can analyze the behavior of similar customers and predict the missing information with high accuracy. According to an IBM study, 25% of data scientists’ time is spent on cleaning and preparing data, but with machine learning, this process can be much more efficient.
2. Personalizing the Customer Experience
Personalization is key to improving customer satisfaction and loyalty. With machine learning, it’s possible to analyze large volumes of customer data, such as purchase history, interactions on social media, and website behavior, to create personalized experiences. Algorithms can identify behavior patterns and individual preferences, offering personalized recommendations in real time.
For example, streaming platforms like Netflix and Spotify use machine learning to analyze user consumption habits and offer recommendations based on that data. This not only enhances the user experience but also increases loyalty and time spent on the platform. According to an Accenture report, companies that use machine learning to personalize the customer experience see a 15% increase in retention.
3. Fraud Detection and Prevention
In sectors like finance and e-commerce, fraud detection and prevention is a priority. Machine learning allows the identification of suspicious patterns in real time by analyzing millions of transactions and user behaviors to detect anomalies that could indicate fraud. Machine learning models can learn from each transaction and adjust their predictions as new data is recorded.
For example, in banking, machine learning algorithms analyze transactions in real time, looking for atypical behaviors such as unauthorized payments or unusual transfers. This allows for the prevention of fraud before it causes significant damage. A Juniper Research study estimates that the implementation of machine learning for fraud prevention will save banks over $200 billion by 2025.
4. Sentiment Analysis on Social Media
Sentiment analysis is a technique used to determine users’ opinions and emotions on social media or online reviews. Machine learning algorithms can automatically analyze large volumes of text, identifying if mentions of a brand or product are positive, negative, or neutral.
For example, a company could use machine learning to analyze customer interactions on Twitter and detect recurring issues in real time, allowing them to improve customer service or adjust their marketing strategy. A Sprout Social report shows that 55% of brands using sentiment analysis improve their communication strategies and customer satisfaction.
Challenges in Implementing Machine Learning in Data Management
While machine learning offers multiple benefits for data management, its implementation may present some challenges. One of the main challenges is data quality. Machine learning algorithms are only as effective as the data they process, so it is essential to ensure that information is clean and structured before training the models.
Another challenge is the lack of skilled personnel. Implementing machine learning in data management requires experts in data science, developers, and analysts who can create and adjust models. Without the right team, it is difficult to fully leverage the capabilities of this technology.
According to a Gartner study, 56% of companies face difficulties finding qualified staff in machine learning and data science. Without skilled personnel, as technology continues to evolve and more accessible tools are developed, this challenge could be reduced in the coming years.
Conclusion
Machine learning is transforming data management, enabling companies to improve data quality, automate processes, and perform more accurate predictive analyzes. From data cleansing to customer experience personalization, this technology offers innovative solutions that enhance decision-making and increase operational efficiency.
Integrating machine learning into your data management strategy will not only improve your business performance but also allow you to maintain a competitive edge in a market increasingly driven by information. The future of analysis is here, and machine learning is the key to unlocking its full potential.
No comment yet, add your voice below!