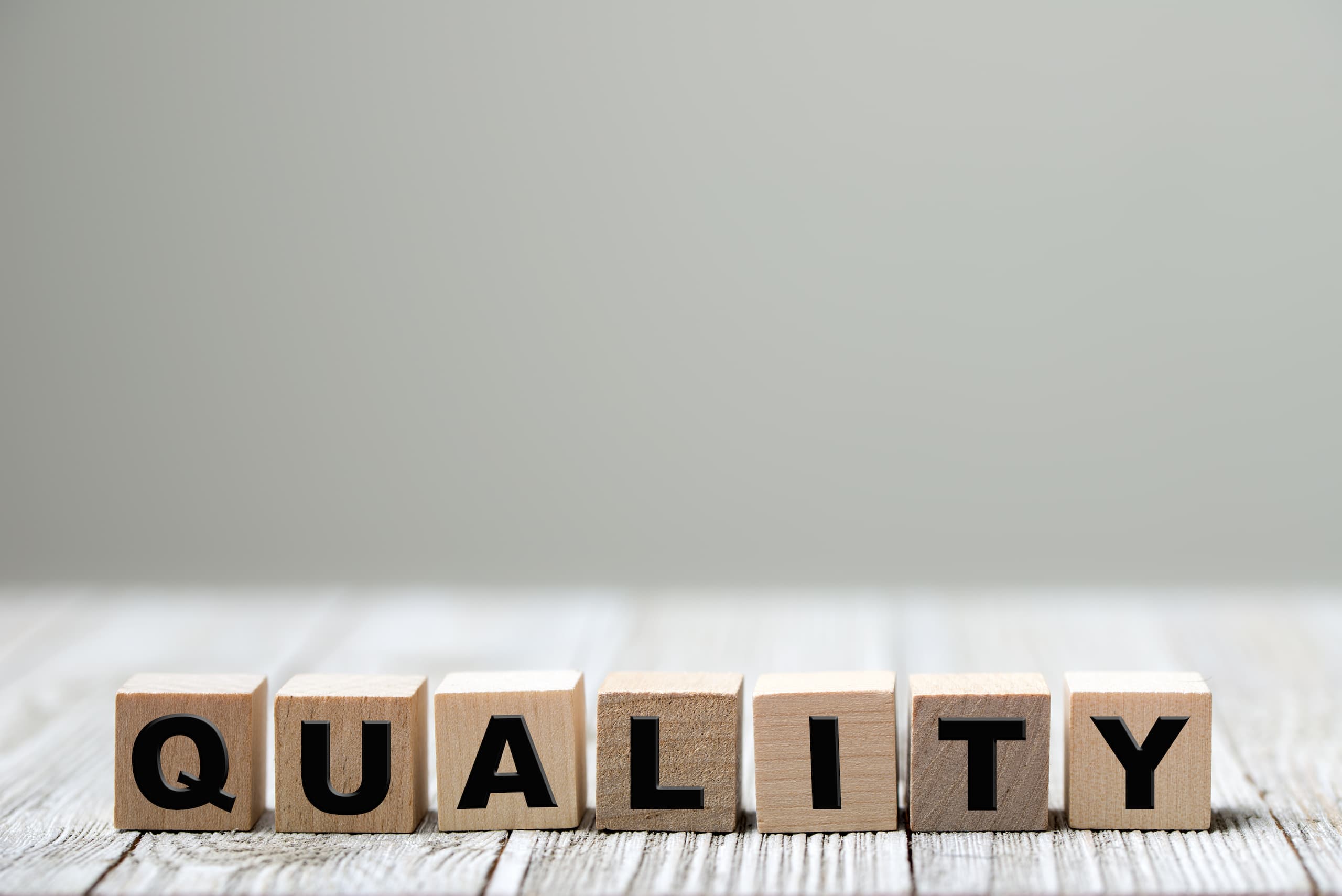
In the digital era, data is one of the most valuable assets for companies. However, the quality of that data is what truly determines success in decision-making and operational efficiency. Having incorrect or incomplete data can lead to costly errors, affect relationships with clients, and reduce competitiveness. For this reason, it is essential to implement an effective data quality audit to ensure that the information on which business decisions are based is accurate and reliable.
In this blog, we will explore key strategies for auditing the quality of business data, ensuring that your organization can maximize the value of its information.
What is a Data Quality Audit?
A data quality audit involves evaluating and analyzing a company’s data to ensure that it meets certain quality standards, such as precision, completeness, consistency, and updating. This audit involves reviewing how data is collected, stored, and managed within the organization’s various systems. The objective is to identify problems or areas for improvement that may be affecting the effective use of the information.
It’s important to remember that low-quality data not only affects operational efficiency but can also impact a company’s reputation and its ability to comply with regulations such as GDPR (General Data Protection Regulation). A regular quality audit is essential to ensure that your data is both accurate and compliant with current regulations.
The Importance of Auditing Data Quality
1. Improves Decision-Making
The quality of the data that a company uses to make decisions can make the difference between success and failure. If the data is inaccurate, incomplete, or outdated, decisions based on this information can be erroneous. A regular data quality audit helps identify and correct these issues, ensuring that the data is reliable.
A Harvard Business Review report found that 47% of companies consider inaccurate data a barrier to effective decision-making. With a proper audit, you can ensure that your team has the correct information at the right time, leading to more informed and effective decisions.
2. Increases Operational Efficiency
When business data is well-managed and of high quality, internal operations become more efficient. Employees don’t have to waste time correcting errors or searching for missing data, and automated processes can run without interruption. By conducting a data quality audit, you can identify weaknesses in your systems and correct them, thereby improving workflow.
According to a Gartner study, companies that improve the quality of their data can increase operational efficiency by 25%. A thorough analysis of data quality allows you to detect and solve problems before they significantly impact the company’s daily operations.
3. Regulatory Compliance
In many industries, compliance with data protection regulations is essential. Regulations such as GDPR or CCPA (California Consumer Privacy Act) require companies to manage data accurately and securely. A data quality audit ensures that information complies with these regulations, avoiding penalties and fines.
For example, GDPR imposes fines of up to 4% of annual revenue on companies that fail to meet data protection requirements. Regularly auditing data quality can help prevent violations by ensuring that all information is properly managed and stored.
Strategies for Auditing Data Quality
1. Establish Clear Data Quality Metrics
Before starting a data quality audit, it’s important to define what “quality” means for your organization. Data quality metrics can vary depending on the industry, but generally include:
- Precision: Do the data reflect reality?
- Completeness: Are any essential data missing?
- Consistency: Are the data consistent across different systems?
- Updating: Are the data up to date?
These metrics allow you to objectively measure data quality and identify which areas need attention. An Experian report highlights that 91% of companies believe that inaccurate data is affecting their ability to fully leverage their information.
2. Implement validation and data cleaning tools
Once you have clear metrics, it’s crucial to use data validation and cleaning tools to detect quality issues. These tools can automate much of the auditing process, identifying common errors, such as duplicates, incorrect values, or inconsistencies across systems.
Tools like Talent or Informatica are known for offering data management and quality solutions that can help conduct automatic audits. These tools not only identify problems but can also suggest or even execute corrections to improve data quality in real-time.
3. Review and Update Your Data Collection Processes
One of the main issues with data quality arises from the moment it is collected. If data collection processes are not rigorous or are poorly designed, it’s likely that the information collected will be inaccurate or incomplete. Conducting an audit also includes reviewing how data are captured, from online forms to manual entry systems.
An Experian study found that 77% of companies believe their data collection processes are ineffective. Reviewing these processes and updating them according to best practices is essential to ensure data quality from the source.
4. Conduct Continuous Data Quality Audits
Data quality auditing should not be a one-time event but rather a continuous practice. As companies collect more data and their systems expand, new quality issues are likely to arise. Establishing a regular audit process, whether monthly or quarterly, ensures that quality issues are detected and resolved before they become serious problems.
According to Deloitte, companies that regularly audit their data quality are 30% more effective in using that information for strategic decision-making. Continuous auditing ensures that data remains accurate, up-to-date, and useful over time.
5. Involve All Departments
Data quality management is not solely the responsibility of the IT department. Since data flows throughout the organization, it is essential that each department is involved in the auditing process. Sales, marketing, finance, and operations teams, for example, should collaborate to identify issues and ensure data is useful for all users.
A PwC report highlights that collaboration between departments improves data quality and enables more efficient information management across the company. Encouraging this collaboration is essential for a successful audit.
Success Stories in Data Quality Auditing
1. Case Study: IBM and the Automation of Data Audits
IBM implemented an automated solution to audit data quality in its global customer management system. By using IBM Watson, the company was able to more quickly identify incorrect, duplicate, or incomplete data, which allowed it to significantly improve the accuracy of its databases. This automated solution reduced 40% of the time dedicated to manual data auditing, improving both efficiency and overall quality.
2. Case Study: Unilever and Data Cleaning in Marketing
Unilever implemented a data quality audit in its marketing department to enhance campaign effectiveness. By analyzing its customer data, the company identified various errors in segmentation and duplicate records. By using auditing tools, Unilever was able to eliminate incorrect data and update its system, resulting in a 20% improvement in customer conversion through personalized campaigns.
Conclusion
A data quality audit is not just a technical task; it is a strategic investment that allows companies to improve their efficiency, make more informed decisions, and comply with data protection regulations. Implementing clear metrics, using validation and cleaning tools, and establishing a continuous auditing process are key steps to ensure that your data is accurate, complete, and up-to-date.
By regularly auditing data quality, you not only avoid operational and regulatory issues but also make the most of valuable information that drives your business growth.
No comment yet, add your voice below!