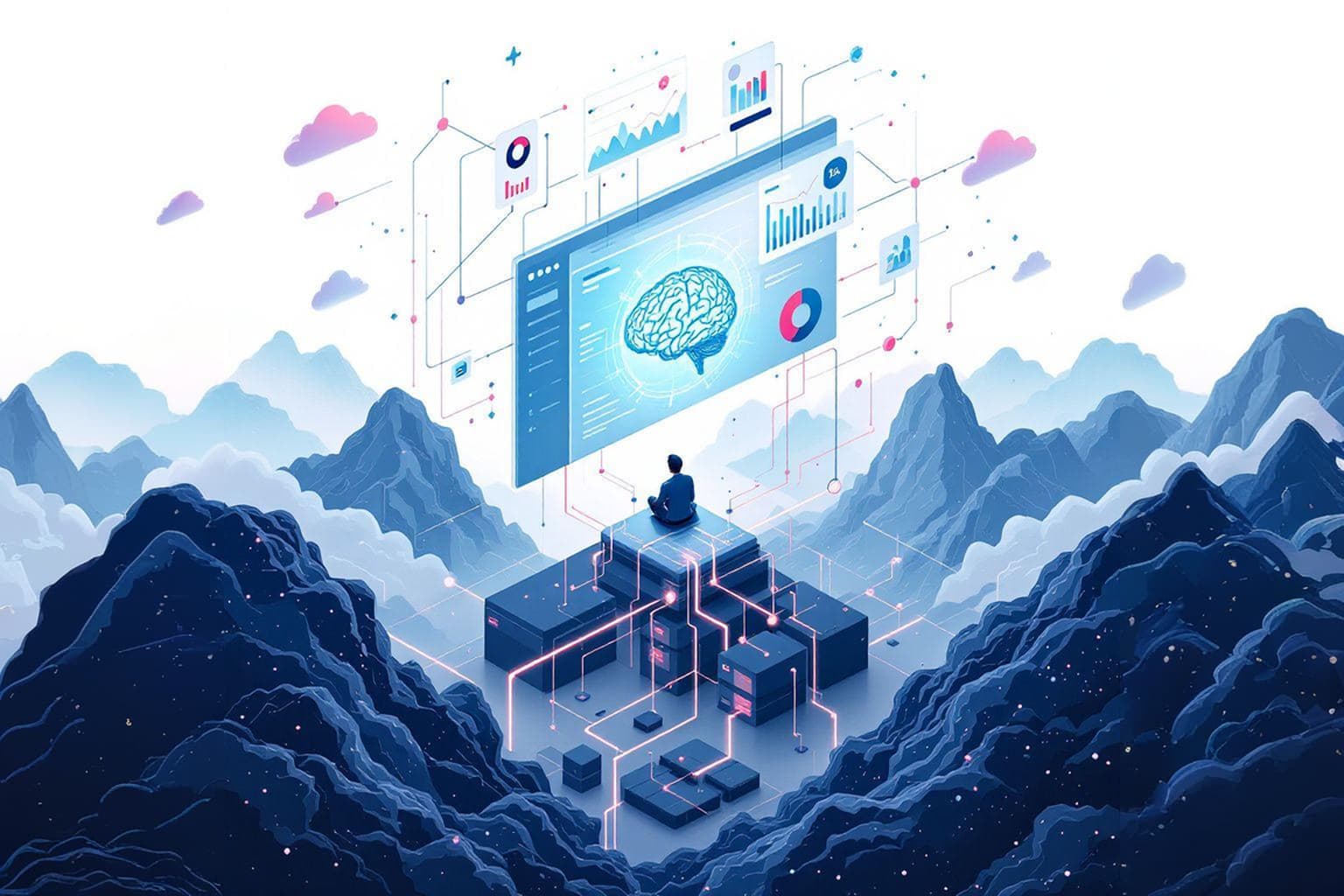
The implementation of predictive models in big data management is a fundamental tool for organizations seeking to understand their operations in depth and make decisions based on complex patterns identified from massive data. These models make it possible to anticipate trends, identify emerging risks and optimize resource allocation by leveraging the vast amount of data that is continuously generated in the digital era.
In this article, we will explore how predictive modeling methodology can be effectively implemented in big data management and the benefits that its adoption brings.
What are predictive models and how do they contribute to big data management?
Predictive models are algorithmic systems designed to analyze historical data to identify patterns and generate predictions about future events. In the context of big data (big data), these models are essential for processing massive information and extracting insights to inform strategic decisions. For example, predictive models make it possible to predict product demand, anticipate problems in the supply chain or model consumer behavior in a granular and accurate way.
In the digital age, organizations generate massive amounts of data on a continuous basis, but the accumulation of data itself does not provide value. The ability to extract relevant information depends on the analysis and processing mechanisms that are applied, and predictive models, together with artificial intelligence and machine learning tools, make it possible to convert these volumes of data into useful information for decision making.
In addition, predictive models make it possible to detect underlying patterns that might go unnoticed by traditional human analysis. For example, an organization might discover that certain customer segments tend to make purchases at certain times of the year, allowing it to tailor marketing strategies and maximize commercial opportunities based on those behaviors.
Benefits of using predictive models on big data
The implementation of predictive models in big data management offers multiple benefits that improve the efficiency and profitability of organizations. Below are some of the main benefits of this technology.
Informed decision making
One of the main benefits of predictive models is the ability to support evidence-based decision making. Analysis of large volumes of data enables companies to gain a clearer understanding of market behavior, product demand and operational performance. This provides a solid foundation for decision makers to act with greater confidence and agility.
For example, an e-commerce company can use predictive models to analyze buying behavior patterns and anticipate demand peaks during specific dates, such as Black Friday. This allows for better inventory planning and resource allocation, avoiding stock-outs or logistical problems. The anticipation of market needs is a significant competitive advantage in a dynamic and competitive environment.
Resource optimization
Another important benefit of predictive modeling is resource optimization. By accurately forecasting what resources will be needed and when, organizations can allocate their assets efficiently, reducing costs and maximizing return on investment.
For example, a logistics company can use predictive modeling to identify when there will be increased demand for its services and plan its routes and personnel accordingly. This approach not only helps reduce costs, but also improves service quality by ensuring adequate availability of resources to meet demand.
Risk identification and mitigation
The early identification of risks is another fundamental benefit of predictive models. These models make it possible to detect patterns that could indicate the existence of potential risks, which facilitates the implementation of preventive measures before problems materialize. This forecasting capability is especially important in industries such as finance, where risks can have considerable implications.
For example, a financial institution might employ predictive models to analyze customer behavior and detect patterns that suggest a potential risk of default. By identifying these risks in advance, the institution can act proactively to mitigate adverse effects by renegotiating credit terms or adjusting loan conditions. This proactive risk management allows organizations to safeguard their interests and minimize negative impact.
Improved customer experience
The use of predictive models also helps to substantially improve the customer experience. By analyzing large volumes of data on consumer behavior, companies can customize product and service offerings to meet the individual needs of each customer. This personalization elevates the quality of the user experience, which fosters customer loyalty and satisfaction.
For example, a streaming platform that uses predictive modeling could analyze a user’s viewing history and suggest content that matches his or her preferences. This level of personalization not only increases user satisfaction, but also increases customer retention by delivering an experience that reflects a deep understanding of consumer preferences.
Product and service innovation
Finally, predictive models foster innovation in products and services. The ability to understand market expectations and consumer preferences enables companies to develop innovative products that better match emerging demand and improve the customer experience.
For example, a technology company can use predictive models to analyze the features consumers value most in their devices, such as battery life or screen size. These insights enable the company to launch products that accurately meet customer expectations, gaining a clear competitive advantage in the marketplace.
Strategies for implementing predictive models on big data.
Clear definition of objectives
Before implementing a predictive model, it is essential to clearly define the objectives to be achieved. Is the intention to forecast demand for a product? Identify customers at risk of churn? Defining the objectives precisely facilitates the selection of the right approach and ensures that efforts are focused on the areas most relevant to the business.
For example, if the objective is to improve customer retention, the predictive model should focus on analyzing behavioral patterns that indicate a risk of churn. On the other hand, if the objective is to optimize logistics, the model should focus on demand, resource availability and distribution route optimization.
Defining objectives also helps determine what data is needed to train the model and how its success will be evaluated, which is essential to ensure that the predictive model brings tangible value to the organization.
Selecting and preparing the right data
In the context of big data, the selection of appropriate data is critical. Not all available data are relevant to every predictive model, so it is essential to identify and select those that have a direct bearing on the problem to be addressed. Data quality is paramount, since inaccurate or irrelevant data can lead to wrong predictions and incorrect decisions.
For example, if the objective is to predict demand for a product, data such as sales history, seasonal trends and market information should be considered. Selecting only relevant data allows the analysis to be more efficient and the predictive model to perform better. Collaborating with business managers is a good practice to determine which data is most relevant.
Data preparation and cleansing
Once the data has been selected, the next step is to prepare and clean the information. This involves removing duplicates, correcting errors and normalizing values. Proper data preparation is critical to ensure that the predictive model works correctly and that predictions are accurate.
For example, inconsistencies in the data, such as incorrect dates or unwarranted outliers, can be misinterpreted by the model and lead to incorrect predictions. Data cleaning, although laborious, is essential to ensure the reliability of the results and the usefulness of the model.
Selection of the appropriate predictive model
Not all predictive models are suitable for all situations. The choice of model depends on the type of problem to be solved and the characteristics of the available data. Regression models, decision trees and neural networks are examples of approaches that may be applicable, depending on the use case.
For example, if the goal is to predict a numerical value, such as sales of a product, a regression model might be the most appropriate. If the objective is to classify customers according to their behavior, a decision tree might be a better choice. The selection of the model should be made considering the specific characteristics of the problem and the nature of the data.
Model validation and fitting
Once the predictive model has been trained, it is crucial to validate it to ensure its effectiveness and accuracy. Validation involves testing the model with a different data set than the one used in training to evaluate its performance and adjust its parameters if necessary. If the model does not reach the expected level of accuracy, it may be necessary to modify its characteristics or, in some cases, to opt for an alternative approach.
Adjustment may involve modifying model parameters, including new data or even selecting a different model if the one initially selected does not meet expectations. The validation and adjustment are fundamental steps to ensure that the predictive model is useful and brings real value to the organization.
Conclusion
The implementation of predictive models in big data management provides organizations with the ability to transform large volumes of data into valuable and actionable information, which facilitates informed decision making. From resource optimization to improved customer experience and product innovation, the benefits are numerous. For effective results, it is crucial to follow a comprehensive strategy that includes clear definition of objectives, proper data selection and preparation, and validation of the predictive model.
If you would like to learn more about how to implement predictive models to optimize big data management in your organization, contact us to explore the most appropriate solutions for your needs.
No comment yet, add your voice below!